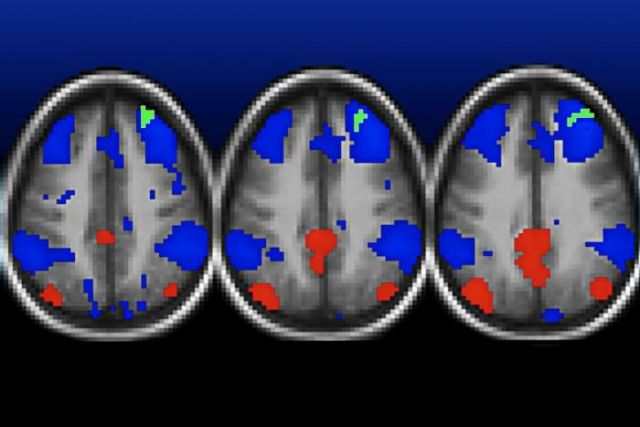
(credit: Deanna Barch)
One of the unfortunate realities of science is that small data sets often produce unreliable results, as any minor, random fluctuations can have a large impact. One solution to this issue has been building ever-larger data sets, where these fluctuations tend to be small compared to any actual effects. One of the notable sources of big data is the UK Biobank; brain scans from people in the Biobank were recently used to identify changes in the brain driven by SARS-CoV-2 infection.
Now, a large team of researchers has turned this idea upside down in a new paper. They took some of the biggest data sets and divided them into smaller pieces to figure out how small data sets could go before things got unreliable. And for at least one type of experiment, the answer is that brain studies need thousands of participants before they’re likely to be reliable. And even then, we shouldn’t expect to see many dramatic effects.
Associate all the things
The research team behind the study termed the type of work they were interested in “brain-wide association studies,” or BWAS. It’s a pretty simple approach. Take a bunch of people and score them for a behavioral trait. Then give them all brain scans and see if any brain structures have differences that consistently correlate with the behavioral trait.